-
Notifications
You must be signed in to change notification settings - Fork 47
Commit
This commit does not belong to any branch on this repository, and may belong to a fork outside of the repository.
- Loading branch information
Showing
1 changed file
with
353 additions
and
0 deletions.
There are no files selected for viewing
This file contains bidirectional Unicode text that may be interpreted or compiled differently than what appears below. To review, open the file in an editor that reveals hidden Unicode characters.
Learn more about bidirectional Unicode characters
Original file line number | Diff line number | Diff line change |
---|---|---|
@@ -0,0 +1,353 @@ | ||
{ | ||
"cells": [ | ||
{ | ||
"cell_type": "markdown", | ||
"metadata": {}, | ||
"source": [ | ||
"## Draw Classification\n", | ||
"\n", | ||
"This interactive notebook demonstrates classification methods with user-generated data. The `drawdata` module allows you to create a data set for classification by sketching the location and density of points. See [Draw Classification](https://apmonitor.com/pds/index.php/Main/DrawClassification) for additional instructions and course content.\n", | ||
"\n", | ||
"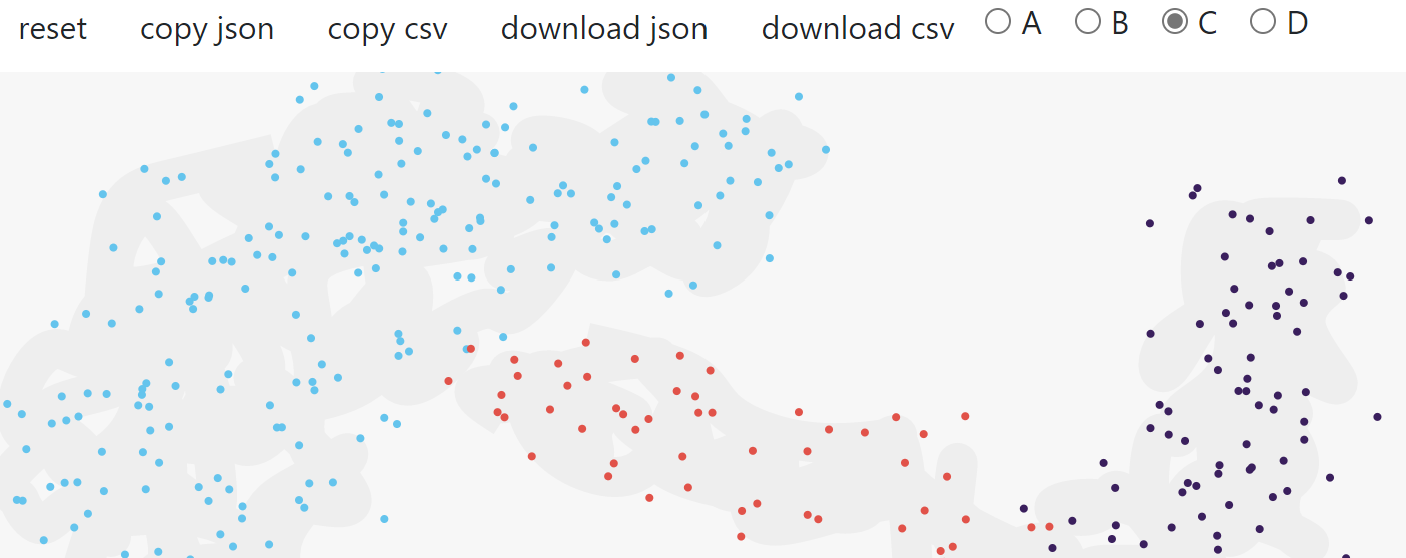\n", | ||
"\n", | ||
"### Install DrawData\n", | ||
"\n", | ||
"Install the `drawdata` module with `pip`. If working in a Jupyter Notebook, a kernel restart is required after the install." | ||
] | ||
}, | ||
{ | ||
"cell_type": "code", | ||
"execution_count": null, | ||
"metadata": { | ||
"scrolled": false | ||
}, | ||
"outputs": [], | ||
"source": [ | ||
"pip install drawdata" | ||
] | ||
}, | ||
{ | ||
"cell_type": "markdown", | ||
"metadata": {}, | ||
"source": [ | ||
"### Create Data\n", | ||
"\n", | ||
"Create a data set with at least two labels such as `A` and `B` by drawing in the window below. Once the data is generated, select `copy csv` to copy the data to the clipboard." | ||
] | ||
}, | ||
{ | ||
"cell_type": "code", | ||
"execution_count": null, | ||
"metadata": { | ||
"scrolled": false | ||
}, | ||
"outputs": [], | ||
"source": [ | ||
"from drawdata import draw_scatter\n", | ||
"draw_scatter()" | ||
] | ||
}, | ||
{ | ||
"cell_type": "markdown", | ||
"metadata": {}, | ||
"source": [ | ||
"### Read Data\n", | ||
"\n", | ||
"[Read the generated data](https://apmonitor.com/pds/index.php/Main/GatherData) with `pandas` and display a random sample of 5 rows. The 5 rows have `x` and `y` location information with the `z` label that is `a`, `b`, `c`, or `d`. " | ||
] | ||
}, | ||
{ | ||
"cell_type": "code", | ||
"execution_count": null, | ||
"metadata": { | ||
"scrolled": true | ||
}, | ||
"outputs": [], | ||
"source": [ | ||
"# select \"copy csv\" before running this cell\n", | ||
"import pandas as pd \n", | ||
"data = pd.read_clipboard(sep=\",\")\n", | ||
"data.sample(5)" | ||
] | ||
}, | ||
{ | ||
"cell_type": "markdown", | ||
"metadata": {}, | ||
"source": [ | ||
"### Describe Data\n", | ||
"\n", | ||
"A [statistical overview](https://apmonitor.com/pds/index.php/Main/StatisticsMath) of the data reveals the number and distribution of points." | ||
] | ||
}, | ||
{ | ||
"cell_type": "code", | ||
"execution_count": null, | ||
"metadata": { | ||
"scrolled": true | ||
}, | ||
"outputs": [], | ||
"source": [ | ||
"data.describe()" | ||
] | ||
}, | ||
{ | ||
"cell_type": "markdown", | ||
"metadata": {}, | ||
"source": [ | ||
"### Visualize Data\n", | ||
"\n", | ||
"Create plots to view data distribution. A classifier creates boundaries to define regions for 2 or more labels. [Data visualization](https://apmonitor.com/pds/index.php/Main/VisualizeData) can give insights on how to build an effective classifier and [identify any data quality issues](https://apmonitor.com/pds/index.php/Main/CleanseData) such as outliers." | ||
] | ||
}, | ||
{ | ||
"cell_type": "code", | ||
"execution_count": null, | ||
"metadata": { | ||
"scrolled": true | ||
}, | ||
"outputs": [], | ||
"source": [ | ||
"import seaborn as sns\n", | ||
"import matplotlib.pyplot as plt\n", | ||
"sns.pairplot(data,hue='z')\n", | ||
"plt.show()" | ||
] | ||
}, | ||
{ | ||
"cell_type": "markdown", | ||
"metadata": {}, | ||
"source": [ | ||
"### Encode Data\n", | ||
"\n", | ||
"[Ordinal number encoding](https://apmonitor.com/pds/index.php/Main/FeatureEngineering) translates text labels (`a`, `b`, `c`, `d`) into numeric labels (`0`, `1`, `2`, `3`). One-hot encoding and feature hashing are two alternative encoding methods." | ||
] | ||
}, | ||
{ | ||
"cell_type": "code", | ||
"execution_count": null, | ||
"metadata": { | ||
"scrolled": true | ||
}, | ||
"outputs": [], | ||
"source": [ | ||
"data['z'] = pd.factorize(data['z'])[0]\n", | ||
"data.sample(10)" | ||
] | ||
}, | ||
{ | ||
"cell_type": "markdown", | ||
"metadata": {}, | ||
"source": [ | ||
"### Scale Data\n", | ||
"\n", | ||
"Many classification methods work best with [scaled data](https://apmonitor.com/pds/index.php/Main/ScaleData). Only the features `x` and `y` are scaled while the label `z` is not scaled to preserve the integer values." | ||
] | ||
}, | ||
{ | ||
"cell_type": "code", | ||
"execution_count": null, | ||
"metadata": { | ||
"scrolled": true | ||
}, | ||
"outputs": [], | ||
"source": [ | ||
"from sklearn.preprocessing import StandardScaler\n", | ||
"s = StandardScaler() # mean=0, standard deviation=1\n", | ||
"dxy = s.fit_transform(data[['x','y']])\n", | ||
"# add scaled values to dataframe\n", | ||
"data['xs'] = dxy[:,0]; data['ys'] = dxy[:,1]\n", | ||
"data.sample(5)" | ||
] | ||
}, | ||
{ | ||
"cell_type": "markdown", | ||
"metadata": {}, | ||
"source": [ | ||
"### Split Data\n", | ||
"\n", | ||
"[Split data](https://apmonitor.com/pds/index.php/Main/SplitData) into train (80%) and test (20%) sets. The test set is to validate the fit created from the training data." | ||
] | ||
}, | ||
{ | ||
"cell_type": "code", | ||
"execution_count": null, | ||
"metadata": { | ||
"scrolled": true | ||
}, | ||
"outputs": [], | ||
"source": [ | ||
"from sklearn.model_selection import train_test_split\n", | ||
"train, test = train_test_split(data, test_size=0.2, shuffle=True)" | ||
] | ||
}, | ||
{ | ||
"cell_type": "markdown", | ||
"metadata": {}, | ||
"source": [ | ||
"### Import Supervised Learning Classifier Packages\n", | ||
"\n", | ||
"Classification: Use supervised learning classification methods:\n", | ||
"\n", | ||
"- Logistic Regression\n", | ||
"- Naïve Bayes\n", | ||
"- Stochastic Gradient Descent\n", | ||
"- K-Nearest Neighbors\n", | ||
"- Decision Tree\n", | ||
"- Random Forest\n", | ||
"- Support Vector Classifier\n", | ||
"- Deep Learning Neural Network\n", | ||
"\n", | ||
"The [Scikit-learn documentation](https://scikit-learn.org/stable/auto_examples/classification/plot_classifier_comparison.html) has additional information on classifiers." | ||
] | ||
}, | ||
{ | ||
"cell_type": "code", | ||
"execution_count": null, | ||
"metadata": { | ||
"scrolled": true | ||
}, | ||
"outputs": [], | ||
"source": [ | ||
"# Import Supervised Learning Classifiers\n", | ||
"from sklearn.linear_model import LogisticRegression # Logistic Regression\n", | ||
"from sklearn.naive_bayes import GaussianNB # Naïve Bayes\n", | ||
"from sklearn.linear_model import SGDClassifier # Stochastic Gradient Descent\n", | ||
"from sklearn.neighbors import KNeighborsClassifier # K-Nearest Neighbors\n", | ||
"from sklearn.tree import DecisionTreeClassifier # Decision Tree\n", | ||
"from sklearn.ensemble import RandomForestClassifier # Random Forest\n", | ||
"from sklearn.svm import SVC # Support Vector Classifier\n", | ||
"from sklearn.neural_network import MLPClassifier # Neural Network" | ||
] | ||
}, | ||
{ | ||
"cell_type": "code", | ||
"execution_count": null, | ||
"metadata": { | ||
"scrolled": true | ||
}, | ||
"outputs": [], | ||
"source": [ | ||
"# Initialize Classifiers\n", | ||
"nb=GaussianNB()\n", | ||
"lr=LogisticRegression()\n", | ||
"sgd=SGDClassifier()\n", | ||
"knn=KNeighborsClassifier()\n", | ||
"dt=DecisionTreeClassifier()\n", | ||
"rfm=RandomForestClassifier()\n", | ||
"svm=SVC()\n", | ||
"nn=MLPClassifier(max_iter=2000)\n", | ||
"\n", | ||
"clsfrs = [[nb,'Naive Bayes'],\n", | ||
" [dt,'Decision Tree'],\n", | ||
" [knn,'K Nearest Neighbors'],\n", | ||
" [svm,'Support Vector Machine'],\n", | ||
" [lr,'Logistic Regression'],\n", | ||
" [sgd,'Stochastic Gradient Descent'],\n", | ||
" [rfm,'Random Forest Classifier'],\n", | ||
" [nn,'Neural Network']\n", | ||
" ]" | ||
] | ||
}, | ||
{ | ||
"cell_type": "markdown", | ||
"metadata": {}, | ||
"source": [ | ||
"### Train Classifiers" | ||
] | ||
}, | ||
{ | ||
"cell_type": "code", | ||
"execution_count": null, | ||
"metadata": { | ||
"scrolled": true | ||
}, | ||
"outputs": [], | ||
"source": [ | ||
"for clf, name in clsfrs:\n", | ||
" clf.fit(train[['x','y']],train['z'])" | ||
] | ||
}, | ||
{ | ||
"cell_type": "markdown", | ||
"metadata": {}, | ||
"source": [ | ||
"### Show Confusion Matrix Result\n", | ||
"\n", | ||
"A confusion matrix shows true positive, false positive, true negative, and false negative groups from the test set. Generate a confusion matrix for each classifier." | ||
] | ||
}, | ||
{ | ||
"cell_type": "code", | ||
"execution_count": null, | ||
"metadata": { | ||
"scrolled": true | ||
}, | ||
"outputs": [], | ||
"source": [ | ||
"from sklearn.metrics import plot_confusion_matrix\n", | ||
"from sklearn.metrics import accuracy_score\n", | ||
"import matplotlib.pyplot as plt\n", | ||
"plt.figure(figsize=(14,7))\n", | ||
"i = 0\n", | ||
"for clf, name in clsfrs:\n", | ||
" i+=1\n", | ||
" ax = plt.subplot(2,4,i)\n", | ||
" plot_confusion_matrix(clf,test[['x','y']],test['z'],ax=ax)\n", | ||
" acc = accuracy_score(test['z'],clf.predict(test[['x','y']]))\n", | ||
" print('{0}: {1:.1f}%'.format(name,acc*100))\n", | ||
" plt.title(name)\n", | ||
"plt.savefig('confusion_matrix.png')" | ||
] | ||
}, | ||
{ | ||
"cell_type": "markdown", | ||
"metadata": {}, | ||
"source": [ | ||
"### Activity\n", | ||
"\n", | ||
"\n", | ||
"\n", | ||
"[AdaBoost (Adaptive Boosting)](https://apmonitor.com/pds/index.php/Main/AdaBoost) is a machine learning algorithm for classification. It is used as a supervisory layer to other classification algorithms such as neural networks, decisions trees, and support vector machines. It takes weak classifiers as a weighted sum and adaptively refines the output to focus on the harder to classify cases.\n", | ||
"\n", | ||
"```python\n", | ||
"from sklearn.ensemble import AdaBoostClassifier\n", | ||
"ab = AdaBoostClassifier()\n", | ||
"ab.fit(train[['x','y']],train['z'])\n", | ||
"yP = ab.predict(test[['x','y']],test['z'])\n", | ||
"```\n", | ||
"\n", | ||
"Train an AdaBoost classifier with the drawn data. Show a confusion matrix and report the accuracy (%)." | ||
] | ||
}, | ||
{ | ||
"cell_type": "code", | ||
"execution_count": null, | ||
"metadata": { | ||
"scrolled": true | ||
}, | ||
"outputs": [], | ||
"source": [] | ||
} | ||
], | ||
"metadata": { | ||
"kernelspec": { | ||
"display_name": "Python 3", | ||
"language": "python", | ||
"name": "python3" | ||
}, | ||
"language_info": { | ||
"codemirror_mode": { | ||
"name": "ipython", | ||
"version": 3 | ||
}, | ||
"file_extension": ".py", | ||
"mimetype": "text/x-python", | ||
"name": "python", | ||
"nbconvert_exporter": "python", | ||
"pygments_lexer": "ipython3", | ||
"version": "3.8.5" | ||
} | ||
}, | ||
"nbformat": 4, | ||
"nbformat_minor": 4 | ||
} |