-
Notifications
You must be signed in to change notification settings - Fork 72
/
cm104.Rmd
754 lines (568 loc) · 29.2 KB
/
cm104.Rmd
1
2
3
4
5
6
7
8
9
10
11
12
13
14
15
16
17
18
19
20
21
22
23
24
25
26
27
28
29
30
31
32
33
34
35
36
37
38
39
40
41
42
43
44
45
46
47
48
49
50
51
52
53
54
55
56
57
58
59
60
61
62
63
64
65
66
67
68
69
70
71
72
73
74
75
76
77
78
79
80
81
82
83
84
85
86
87
88
89
90
91
92
93
94
95
96
97
98
99
100
101
102
103
104
105
106
107
108
109
110
111
112
113
114
115
116
117
118
119
120
121
122
123
124
125
126
127
128
129
130
131
132
133
134
135
136
137
138
139
140
141
142
143
144
145
146
147
148
149
150
151
152
153
154
155
156
157
158
159
160
161
162
163
164
165
166
167
168
169
170
171
172
173
174
175
176
177
178
179
180
181
182
183
184
185
186
187
188
189
190
191
192
193
194
195
196
197
198
199
200
201
202
203
204
205
206
207
208
209
210
211
212
213
214
215
216
217
218
219
220
221
222
223
224
225
226
227
228
229
230
231
232
233
234
235
236
237
238
239
240
241
242
243
244
245
246
247
248
249
250
251
252
253
254
255
256
257
258
259
260
261
262
263
264
265
266
267
268
269
270
271
272
273
274
275
276
277
278
279
280
281
282
283
284
285
286
287
288
289
290
291
292
293
294
295
296
297
298
299
300
301
302
303
304
305
306
307
308
309
310
311
312
313
314
315
316
317
318
319
320
321
322
323
324
325
326
327
328
329
330
331
332
333
334
335
336
337
338
339
340
341
342
343
344
345
346
347
348
349
350
351
352
353
354
355
356
357
358
359
360
361
362
363
364
365
366
367
368
369
370
371
372
373
374
375
376
377
378
379
380
381
382
383
384
385
386
387
388
389
390
391
392
393
394
395
396
397
398
399
400
401
402
403
404
405
406
407
408
409
410
411
412
413
414
415
416
417
418
419
420
421
422
423
424
425
426
427
428
429
430
431
432
433
434
435
436
437
438
439
440
441
442
443
444
445
446
447
448
449
450
451
452
453
454
455
456
457
458
459
460
461
462
463
464
465
466
467
468
469
470
471
472
473
474
475
476
477
478
479
480
481
482
483
484
485
486
487
488
489
490
491
492
493
494
495
496
497
498
499
500
501
502
503
504
505
506
507
508
509
510
511
512
513
514
515
516
517
518
519
520
521
522
523
524
525
526
527
528
529
530
531
532
533
534
535
536
537
538
539
540
541
542
543
544
545
546
547
548
549
550
551
552
553
554
555
556
557
558
559
560
561
562
563
564
565
566
567
568
569
570
571
572
573
574
575
576
577
578
579
580
581
582
583
584
585
586
587
588
589
590
591
592
593
594
595
596
597
598
599
600
601
602
603
604
605
606
607
608
609
610
611
612
613
614
615
616
617
618
619
620
621
622
623
624
625
626
627
628
629
630
631
632
633
634
635
636
637
638
639
640
641
642
643
644
645
646
647
648
649
650
651
652
653
654
655
656
657
658
659
660
661
662
663
664
665
666
667
668
669
670
671
672
673
674
675
676
677
678
679
680
681
682
683
684
685
686
687
688
689
690
691
692
693
694
695
696
697
698
699
700
701
702
703
704
705
706
707
708
709
710
711
712
713
714
715
716
717
718
719
720
721
722
723
724
725
726
727
728
729
730
731
732
733
734
735
736
737
738
739
740
741
742
743
744
745
746
747
748
749
750
751
752
753
754
# (4) Functional programming in R: Part II
```{r include=FALSE}
knitr::opts_chunk$set(echo=TRUE, warning=FALSE, message=FALSE)
```
```{r, warning = FALSE, error = FALSE}
library(tidyverse)
library(rlang)
library(glue)
#install.packages('docopt')
#install.packages('testthat')
library(docopt) # you will need to install this using install.packages('docopt')
library(testthat) # you will need to install this using install.packages('testthat')
```
## Today's Agenda
- Announcements:
- If you need Git help, please come to our [office hours](https://stat545.stat.ubc.ca/officehours/)! This is a crucial concept and it's worth getting it right!
- Your project repos should be *public*, assignment and participation repos are *private*
- Apologies if I mis-spoke and for any confusion. See [GitHub issue](https://github.com/STAT547-UBC-2019-20/Discussions/issues/17) for more
- Next Thursday's class
- Part 1: Running Rscripts from the terminal/command prompt (20 mins)
- "REPL" vs. non-interactive scripts
- Converting interactive R code to a scripted, non-interactive version
- Part 2: Command-line arguments and docopt (30 mins)
- Motivating command-line arguments
- Options for specifying command-line arguments
- Adding command-line arguments to your R scripts
- Part 3: Writing tests for your functions (25 mins)
- Motivating the use of tests in programming
- Introducing the `testthat` package
- Writing tests using the `testthat` package
## Learning outcomes for this lecture
1. Evaluate when it is optimal to code in a REPL framework (interactively) vs. using scripts.
1. Demonstrate the ability to run interactive R code from a .R script.
1. Use principles of functional programming to create functions inside R scripts.
1. Explain the purpose of command-line arguments and describe the various flavours (optional, required, long and short forms, repeating, etc...).
1. Use the `docopt` to create commandline arguments for R scripts.
1. Defend the use of tests when programming.
1. Use the `testthat` library to write tests for R functions.
## Part 1: Running Rscripts from the terminal/command prompt (15 mins)
This section has been adapted from Tiffany Timbers' DSCI 522 lectures found [here](https://github.com/UBC-MDS/DSCI_522_dsci-workflows/blob/master/lectures/02_lecture-intro-to-scripts.ipynb).
### "REPL" vs. non-interactive scripts
So far in STAT 545 and 547M, we have only been working on Rmd files and interactively working with code.
We run and re-run specific code chunks, add bits of code, and re-run it to get updated results.
Of course, this works - but is it reproducible ?
Can you trust that in a week, or a month, or a year from now that you will be able to reproduce the exact steps needed to get the same answer?
Usually not.
The above style of working actually has a name: REPL, or Read-Evaluate-Print-Loop.
Despite the fact that it's usually not easily reproducible, it is actually VERY useful!
It's good for:
- solving smaller problems in projects limited in scope
- explore and play around with unfamiliar and unknown datasets
- developing code that will eventually make its way into a script or turned into a function
- developing code that you will only use once
It is rare that data scientists would go directly towards creating scripts and functions without first developing them in a REPL environment.
At this point, you may be wondering what scripts *are* and *why* we should write them?.
Well, an R script is just a text file that contains R code that is run in a sequence, usually with no or minimal intervention from the user.
Scripts are generally run from the Terminal, but can also be run from IDEs (interactive developer environment; RStudio is an IDE) and even the Rconsole using `source(script_name.R)`.
As for why:
- Scripts are generally more time efficient (especially for long term usage)
- You can combine multiple scripts to create an analysis pipeline (next week!)
- Scripts can be reused and adapted for different purposes; additional functionality can be added to scripts without removing the ability of it working for previous analyses
- Are an important component of reproducible workflows
Alright now that we know how REPL, or interactive scripts differ from non-interactive scripts, let's move on and actually create R scripts from interactive R code.
### Converting interactive R code to a scripted, non-interactive version
Let's just do a quick sanity check to make sure our script can run.
- Step 1: In the cm104 directory, create a new blank .R script and save it as `first_script.R`
- In RStudio, File > New File > R Script.
- Step 2: Write the following R code inside `first_script.R`:
- `print('Hello World')`
- Step 3: Run the Rscript by opening a new Terminal, navigating to the directory where `first_script.R` exists and then type:
- `Rscript first_script.R`
- "Hello World" should be printed to the Terminal and the R script will finish.
- Step 4: Navigate to one directory above the current directory with `cd ..`
- Now try running `first_script.R` - does it work?
- How would you modify `Rscript first_script.R` to make it work (without going into the `cm104` directory)?
```
## YOUR SOLUTION HERE
Rscript cm104/first_script.R
```
Okay, let's do something slightly more interesting: a quick demo using an interactive script that we're going to write together.
#### Your Task 1:
- Start with the `mtcars` dataset
- Use `dplyr::mutate` to create a new column for the fuel efficiency in L/100km rather than mpg
- Create a plot of Horse power vs. fuel efficiency
```{r interactive_mtcars_demo}
head(mtcars)
## YOUR SOLUTION HERE
mtcars %>%
mutate(lkm = 235.2145 / mpg) %>% # imperial = 282.48, #US = 235.21
ggplot(aes(x = lkm, y = hp)) + geom_point() +
theme_bw(16) + # Set font size like this
labs(x = 'Fuel efficiency (L/100 km)',
y = 'Horse power (hp)',
title = "Fuel efficiency by horse power")
```
#### Your Task 2:
- Use the code above as a starting point
- Copy the code above and paste it into `first_script.R`
- Move the mutate command into a separate function
- Your function should take in a string argument with two options: "USA" and "Imperial" (set a reasonable default)
- Try running the script. Did it work? Was there a plot produced?
- If yes, great! If not, what did you have to change to get it to work?
- Can you explain why?
```{r working_mtcars_demo}
## YOUR SOLUTION HERE
library(tidyr)
library(dplyr)
library(ggplot2)
fuel_conversion <- function (df, gallon_type = 'USA') {
if (gallon_type == 'USA') {
conv = 235.2145
} else {
conv = 282.48
}
df %>% mutate(lkm = conv / mpg)
}
mtcars %>%
fuel_conversion(gallon_type = 'Imperial') %>%
ggplot(aes(x = lkm, y = hp)) + geom_point() +
theme_bw(16) + # Set font size like this
labs(x = 'Fuel efficiency (L/100 km)',
y = 'Horse power (hp)',
title = "Fuel efficiency by horse power") +
ggsave('test_plot.png', width = 8, height = 5)
```
Great!
Looks like things are working!
Let's organize our script in a slightly more useful way.
### Structure of scripts
It is very good practice to organize the code in your script into a main function and other functions.
This practice keeps your code readable and organized, this has some additional benefits we will discuss later.
Here is a suggested script organization:
```
# documentation for how to use scripts and comments
# Load libraries and packages
# parse/define command line arguments here
# define main function
main <- function(){
# code for "guts" of script goes here
}
# Code for other functions & tests
# call main function
main()
```
For documentation, it is suggested to use `roxygen2` [style](https://cran.r-project.org/web/packages/roxygen2/vignettes/roxygen2.html).
Here is an example of this style:
```
#' Add together two numbers
#'
#' @param x A number
#' @param y A number
#' @return The sum of \code{x} and \code{y}
#' @examples
#' add(1, 1)
#' add(10, 1)
add <- function(x, y) {
x + y
}
```
#### Your Task 3: Create a script and conform to the suggested structure
Let's edit our script from the previous section (`first_script.R`) to conform to this structure.
When you're done, paste in the contents of your script here:
```
## YOUR SOLUTION HERE (contents of first_script.R)
# author: Firas Moosvi
# date: 2020-03-05
"This script adds a new column to the `mtcars` dataset converting fuel efficiency from miles per gallon to Litres per 100km. This script takes the gallon_type as the variable argument.
Usage: first_script.R <gallon_type>
"
library(tidyr)
library(dplyr)
library(ggplot2)
library(glue)
main <- function(gallon_type){
mtcars %>%
fuel_conversion(gallon_type = 'Imperial') %>%
ggplot(aes(x = lkm, y = hp)) + geom_point() +
theme_bw(16) + # Set font size like this
labs(x = 'Fuel efficiency (L/100 km)',
y = 'Horse power (hp)',
title = "Fuel efficiency by horse power") +
ggsave('test_plot.png', width = 8, height = 5)
print(glue("The gallon_type is ", gallon_type))
}
#' calculate fuel efficiency in L/100km
#'
#' @param df is an input of the mtcars dataframe
#' @param gallon_type is a string either `USA` or `Imperial`
#' @examples
#' fuel_conversion('USA')
fuel_conversion <- function (df, gallon_type = 'USA') {
if (gallon_type == 'USA') {
conv = 235.2145
} else {
conv = 282.48
}
df %>% mutate(lkm = conv / mpg)
}
### tests
main()
```
### Summary and key points of scripts
- ## YOUR NOTES HERE
-
-
What if we wanted to specify the arguments of assignment to the imperial definition of gallon rather than the US one?
For that, we need to look at command line arguments!
## Part 2: Command-line arguments and docopt (30 mins)
This section has been adapted from Tiffany Timbers' DSCI 522 lectures found [here](https://github.com/UBC-MDS/DSCI_522_dsci-workflows/blob/master/lectures/02_lecture-intro-to-scripts.ipynb).
### Introduction to docopt
We will be following the documentation for docopt [here](https://docopt.org).
Key points:
- Arguments for your script can be specified by "position" or as "options"
- Options is almost always better as it allows your code and scripts to be more readable
- To allow your non-mandatory arguments use `[ ]`
- Use parantheses `( )` for required arguments
- Complex arguments can be set up using the `|` (pipe) indicating "OR" as well as `...` to indicate repeating elements
### Adding command line arguments in R
Let's make our script more flexible, and specify when we call the script, whether the `gallon_type` is `US` or `Imperial` variable.
To do this, we use the `docopt` R package. This will allow us to collect the text we enter at the command line when we call the script, and make it available to us when we run the script.
When we run `docopt` it takes the text we entered at the command line and gives it to us as a named list of the text provided after the script name.
The names of the items in the list come from the documentation.
Whitespace at the command line is what is used to parse the text into separate items in the vector.
```
# author: Firas Moosvi
# date: 2020-03-05
"This script adds a new column to the `mtcars` dataset converting fuel efficiency from miles per gallon to Litres per 100km. This script takes the gallon_type as the variable argument.
Usage: first_script.R <gallon_type>
" -> doc # THIS IS NEW
library(tidyr)
library(dplyr)
library(ggplot2)
library(docopt) # THIS IS NEW
library(glue)
# THIS IS NEW
opt <- docopt(doc) # This is where the "Usage" gets converted into code that allows you to use commandline arguments
main <- function(gallon_type){
mtcars %>%
fuel_conversion(gallon_type = 'Imperial') %>%
ggplot(aes(x = lkm, y = hp)) + geom_point() +
theme_bw(16) + # Set font size like this
labs(x = 'Fuel efficiency (L/100 km)',
y = 'Horse power (hp)',
title = "Fuel efficiency by horse power") +
ggsave('test_plot.png', width = 8, height = 5)
print(glue("The gallon_type is ", gallon_type))
}
#' calculate fuel efficiency in L/100km
#'
#' @param df is an input of the mtcars dataframe
#' @param gallon_type is a string either `USA` or `Imperial`
#' @examples
#' fuel_conversion('USA')
fuel_conversion <- function (df, gallon_type = 'USA') {
if (gallon_type == 'USA') {
conv = 235.2145
} else {
conv = 282.48
}
df %>% mutate(lkm = conv / mpg)
}
### tests
main(opt$gallon_type) # THIS IS NEW
```
To run this script from the commandline, we would open a Terminal, navigate to the directory containing the script, and run:
```
Rscript first_script.R 'Imperial'
```
And that's it!
You've just written your first R script with command line options!
This is a big deal!
The power you now wield is enormous!
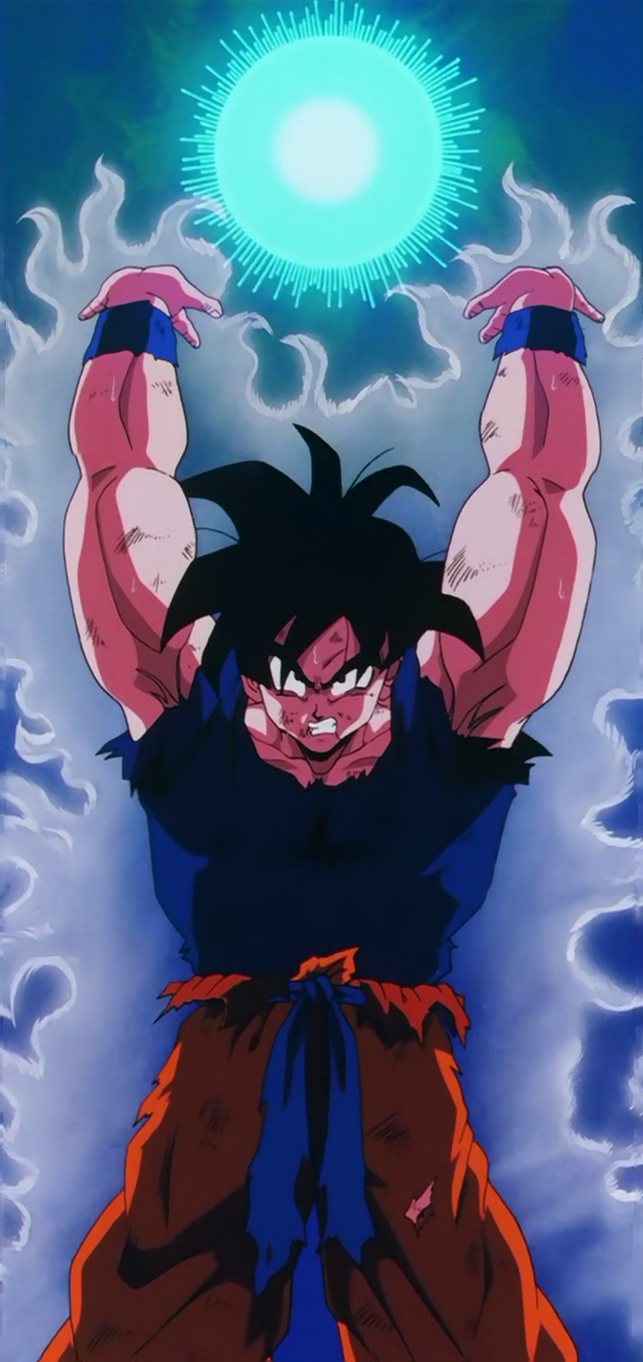
Image obtained from Dragonball fandom [here](https://dragonball.fandom.com/wiki/Spirit_Bomb?file=Goku_DBZ_Ep_93_007.png).
Original copyright belongs to Dragon Ball Z © 2003 Bird Studio / Shueisha, Toei Animation. Licensed by FUNimation® Productions, Ltd. All Rights Reserved. Dragon Ball Z and all logos, character names and distinctive likenesses thereof are trademarks of TOEI ANIMATION.
### Positional vs. option arguments
In the examples above, we used `docopt` to specify a positional argument (gallon_type).
This means that the order matters!
If we added another argument the same way we added `gallon_type`, the order that we specify the arguments will matter.
Our script will likely throw an error because it will try to perform the wrong operations using the wrong arguments.
Another downside to positional arguments, is that without good documentation, they can be less readable.
And certainly the call to the script to is less readable.
We should instead give the arguments names using `--ARGUMENT_NAME` syntax.
We call these "options".
Below is the same script but specified using options rather than to positional arguments:
```
# author: Firas Moosvi
# date: 2020-03-05
"This script adds a new column to the `mtcars` dataset converting fuel efficiency from miles per gallon to Litres per 100km. This script takes the gallon_type as the variable argument.
Usage: first_script.R --gallon_type=<gallon_type>
" -> doc
library(tidyr)
library(dplyr)
library(ggplot2)
library(docopt)
library(glue)
opt <- docopt(doc) # This is where the "Usage" gets converted into code that allows you to use commandline arguments
main <- function(gallon_type){
mtcars %>%
fuel_conversion(gallon_type = 'Imperial') %>%
ggplot(aes(x = lkm, y = hp)) + geom_point() +
theme_bw(16) + # Set font size like this
labs(x = 'Fuel efficiency (L/100 km)',
y = 'Horse power (hp)',
title = "Fuel efficiency by horse power") +
ggsave('test_plot.png', width = 8, height = 5)
print(glue("The gallon_type is ", gallon_type))
}
#' calculate fuel efficiency in L/100km
#'
#' @param df is an input of the mtcars dataframe
#' @param gallon_type is a string either `USA` or `Imperial`
#' @examples
#' fuel_conversion('USA')
fuel_conversion <- function (df, gallon_type = 'USA') {
if (gallon_type == 'USA') {
conv = 235.2145
} else {
conv = 282.48
}
df %>% mutate(lkm = conv / mpg)
}
### tests
main(opt$gallon_type)
```
Now we would call the script like this:
```
Rscript first_script.R --gallon_type='Imperial'
```
Now that the arguments have names, if we had multiple arguments, the order doesn't matter anymore!
#### Your Task 4: Update the script above and add a second required argument and print it out using glue.
```
## YOUR SOLUTION HERE (contents of first_script.R)
```
### Summary and key points of options
- `docopt` is a REALLY useful R package that allows us to easily add arguments and options to our scripts
- You should always have named arguments, or options for scripts; makes your scripts much more readable
- Arguments for your script can be specified by "position" or as "options"
- Options is almost always better as it allows your code and scripts to be more readable
- To allow your non-mandatory arguments use `[ ]`
- Use parantheses `( )` for required arguments
- Complex arguments can be set up using the `|` (pipe) indicating "OR" as well as `...` to indicate repeating elements
## Quarter pole course check-in
Now that we're roughly a quarter way through the course, I thought I'd check in to see how things are going.
Please fill out this very quick Stop-Start-Continue [here](https://firasmoosvi.typeform.com/to/KvvsII) so that I know what's going well, what needs to be improved, and what requires immediate intervention.
## Part 3: Writing tests for your functions
This section has been adapted from
This section is adapted from Chapter 8 of the [tidynomicon textbook by Greg Wilson](https://gvwilson.github.io/tidynomicon/).
### Testing and Error Handling
Novices write code and pray that it works.
Experienced programmers know that prayer alone is not enough, and take steps to protect what little sanity they have left.
This chapter looks at the tools R gives us for doing this.
### How does R handle errors?
We say that the operation [signals](glossary.html#signal-condition) a [condition](glossary.html#condition) that some other piece of code then [handles](glossary.html#handle-condition).
These things are all simpler to do using the rlang library, so we begin by loading that:
In order of increasing severity, the three built-in kinds of conditions are [messages](glossary.html#message), [warnings](glossary.html#warning), and [errors](glossary.html#error).
(There are also interrupts, which are generated by the user pressing Ctrl-C to stop an operation, but we will ignore those for the sake of brevity.)
We can signal conditions of these kinds using the functions `message`, `warning`, and `stop`, each of which takes an error message as a parameter:
```{r message-warning-error, error=TRUE}
message("This is a message.")
warning("This is a warning.\n")
stop("This is an error.")
```
Note that we have to supply our own line ending for warnings but not for the other two cases.
Note also that there are very few situations in which a warning is appropriate: if something has truly gone wrong then we should stop, but otherwise we should not distract users from more pressing concerns.
The bluntest of instruments for handling errors is to ignore them.
If a statement is wrapped in the function `try` then errors that occur in it are still reported, but execution continues.
Compare this:
```{r attempt-without-try, error=TRUE}
attemptWithoutTry <- function(left, right){
temp <- left + right
"result" # returned
}
result <- attemptWithoutTry(1, "two")
cat("result is", result)
```
with this:
```{r attempt-using-try}
attemptUsingTry <- function(left, right){
temp <- try(left + right)
"value returned" # returned
}
result <- attemptUsingTry(1, "two")
cat("result is", result)
```
We can suppress error messages from `try` by setting `silent` to `TRUE`:
```{r attempt-quietly}
attemptUsingTryQuietly <- function(left, right){
temp <- try(left + right, silent = TRUE)
"result" # returned
}
result <- attemptUsingTryQuietly(1, "two")
cat("result is", result)
```
Do NOT do this, lest you one day find yourself lost in a silent hellscape.
Should you more sensibly wish to handle conditions rather than ignore them, you may invoke `tryCatch`.
We begin by raising an error explicitly:
```{r r-try-catch}
tryCatch(
stop("our message"),
error = function(cnd) print(glue("error object is {cnd}"))
)
```
We can now run a function that would otherwise blow up:
```{r r-try-catch-triggered}
tryCatch(
attemptWithoutTry(1, "two"),
error = function(cnd) print(glue("error object is {cnd}"))
)
```
### What should I know about testing in general?
In keeping with common programming practice, we have left testing until the last possible moment.
The standard testing library for R is `testthat` and this is how tests are handled in R:
1. Each test consists of a single function that tests a single property or behavior of the system.
2. Tests are collected into files with prescribed names that can be found by a test runner (code that is automatically run once after each unit test).
3. Shared setup (code that is automatically run once before each unit test) and teardown (Code that is automatically run once after each unit test) steps are put in functions of their own.
For now in STAT547, we will use the `testthat` library to test our functions in-line our Rscripts, though it is much better practice to set up your tests in a `tests` directory and have them run automatically.
Let's load it and write our first test:
```{r introduce-testthat}
test_that("Zero equals itself", {expect_equal(0, 0)})
```
As is conventional with unit testing libraries, no news is good news: if a test passes, it doesn't produce output because it doesn't need our attention.
Let's try something that ought to fail:
```{r error = TRUE}
test_that("Zero equals one", {expect_equal(0, 1)})
```
If you run this, you should see something like:
```
Error: Test failed: 'Zero equals one' * 0 not equal to 1. 1/1 mismatches [1] 0 - 1 == -1
```
Good: we can draw some comfort from the fact that Those Beyond have not yet changed the fundamental rules of arithmetic.
But what are the curly braces around `expect_equal` for? The answer is that they create a code block for `test_that` to run.
We can run `expect_equal` on its own:
```{r expect-equal-alone, error=TRUE}
expect_equal(0, 1)
```
but that doesn't produce a summary of how many tests passed or failed.
Passing a block of code to `test_that` also allows us to check several things in one test:
```{r pass-code-block, error=TRUE}
test_that("Testing two things", {
expect_equal(0, 0)
expect_equal(0, 1)
})
```
A block of code is *not* the same thing as an anonymous function, which is why running this block of code does nothing — the "test" defines a function
but doesn't actually call it:
```{r anonymous-function}
test_that("Using an anonymous function", function() {
print("In our anonymous function")
expect_equal(0, 1)
})
```
### How should I organize my tests?
Running blocks of tests by hand is a bad practice.
Instead, we should put related tests in files and then put those files in a directory called `tests/testthat`.
We can then run some or all of those tests with a single command.
To start, create in your `cm104` folder, create this file: `./tests/testthat/test_example.R`:
```
library(testthat)
context("Demonstrating the testing library")
test_that("Testing a number with itself", {
expect_equal(0, 0)
expect_equal(-1, -1)
expect_equal(Inf, Inf)
})
test_that("Testing different numbers", {
expect_equal(0, 1)
})
test_that("Testing with a tolerance", {
expect_equal(0, 0.01, tolerance = 0.05, scale = 1)
expect_equal(0, 0.01, tolerance = 0.005, scale = 1)
})
```
The first line loads the testthat package, which gives us our tools.
The call to `context` on the second line gives this set of tests a name for reporting purposes.
After that, we add as many calls to `test_that` as we want, each with a name and a block of code.
We can now run this file from within RStudio:
```
test_dir("tests/testthat")
```
The output should be:
```
✔ | OK F W S | Context
⠏ | 0 | Skipping rows correctly
✖ | 0 5 | Skipping rows correctly
───────────────────────────────────────────────────────────────────────────
test_determine_skip_rows_a.R:9: failure: The right row is found when there are header rows
`result` not equal to 2.
Lengths differ: 0 is not 1
test_determine_skip_rows_a.R:14: failure: The right row is found when there are header rows and blank lines
`result` not equal to 3.
Lengths differ: 0 is not 1
test_determine_skip_rows_a.R:19: failure: The right row is found when there are no header rows to discard
`result` not equal to 0.
Lengths differ: 0 is not 1
test_determine_skip_rows_a.R:23: failure: No row is found when 'iso3' isn't present
`determine_skip_rows("a1,a2\nb1,b1\n")` did not throw an error.
test_determine_skip_rows_a.R:28: failure: No row is found when 'iso3' is in the wrong place
`determine_skip_rows("stuff,iso3\n")` did not throw an error.
───────────────────────────────────────────────────────────────────────────
⠏ | 0 | Skipping rows correctly
✔ | 5 | Skipping rows correctly
⠏ | 0 | Demonstrating the testing library
✖ | 4 2 | Demonstrating the testing library
───────────────────────────────────────────────────────────────────────────
test_example.R:11: failure: Testing different numbers
0 not equal to 1.
1/1 mismatches
[1] 0 - 1 == -1
test_example.R:16: failure: Testing with a tolerance
0 not equal to 0.01.
1/1 mismatches
[1] 0 - 0.01 == -0.01
───────────────────────────────────────────────────────────────────────────
⠏ | 0 | Finding empty rows
✖ | 1 2 | Finding empty rows
───────────────────────────────────────────────────────────────────────────
test_find_empty_a.R:9: failure: A single non-empty row is not mistakenly detected
`result` not equal to NULL.
Types not compatible: integer is not NULL
test_find_empty_a.R:14: failure: Half-empty rows are not mistakenly detected
`result` not equal to NULL.
Types not compatible: integer is not NULL
───────────────────────────────────────────────────────────────────────────
⠏ | 0 | Finding empty rows
✔ | 3 | Finding empty rows
⠏ | 0 | Testing properties of tibbles
✔ | 1 1 | Testing properties of tibbles
───────────────────────────────────────────────────────────────────────────
test_tibble.R:6: warning: Tibble columns are given the name 'value'
`as.tibble()` is deprecated, use `as_tibble()` (but mind the new semantics).
This warning is displayed once per session.
───────────────────────────────────────────────────────────────────────────
══ Results ════════════════════════════════════════════════════════════════
Duration: 0.2 s
OK: 14
Failed: 9
Warnings: 1
Skipped: 0
```
Care is needed when interpreting these results.
There are four `test_that` calls, but eight actual checks, and the number of successes and failures is counted by recording the latter, not the former.
What then is the purpose of `test_that`?
Why not just use `expect_equal` and its kin, such as `expect_true`, `expect_false`, `expect_length`, and so on?
The answer is that it allows us to do one operation and then check several things afterward.
Our tests still aren't checking anything statistical, but without trustworthy data,
our statistics will be meaningless.
Tests like these allow our future selves to focus on making new mistakes instead of repeating old ones.
### What type of tests can I do with `testthat` ?
[This page](https://testthat.r-lib.org/reference/index.html) has a thorough list of "expectations".
Some useful functions are:
- test_dir(), test_package(), test_check(), is_testing(), testing_package(): Expectation: is returned value less or greater than specified value?
- expect_equal(), expect_equivalent(), expect_identical(), expect_reference(): Expectation: is the object equal to a value?
- expect_length(): Expectation: does a vector have the specified length?
... etc
### More practice with `test_that`
The [next two sub-sections](http://tidynomicon.tech/testerror.html#how-should-i-organize-my-tests) of the tidynomicon chapter on testing are likely beyond the scope of STAT547, but I highly encourage you to go through on your own time when you're about to embark on a project for your research!
### Summary and key points of testing
- The three built-in levels of conditions are messages, warnings, and errors.
- Programs/scripts can signal these themselves using the functions `message`, `warning`, and `stop`.
- Operations can be placed in a call to the function tryCatch to handle errors.
- Use `testthat` to write unit tests for R.
- Put tests in files called test_group.R and call them test_something.
- Write tests for data transformation steps (if you can) as well as library functions.
## Additional Resources
- Stat545.com by Jenny Bryan - [Tests](https://stat545.com/functions-part3.html)
- Wickham and Bryan's R Packages book - [Testing chapter](https://r-pkgs.org/tests.html)
- Tidynomicon by Greg Wilson - [Tests in R]