-
Notifications
You must be signed in to change notification settings - Fork 798
Commit
This commit does not belong to any branch on this repository, and may belong to a fork outside of the repository.
Move sam2 notebooks to separate places
- Loading branch information
Showing
14 changed files
with
734 additions
and
146 deletions.
There are no files selected for viewing
This file contains bidirectional Unicode text that may be interpreted or compiled differently than what appears below. To review, open the file in an editor that reveals hidden Unicode characters.
Learn more about bidirectional Unicode characters
This file contains bidirectional Unicode text that may be interpreted or compiled differently than what appears below. To review, open the file in an editor that reveals hidden Unicode characters.
Learn more about bidirectional Unicode characters
This file contains bidirectional Unicode text that may be interpreted or compiled differently than what appears below. To review, open the file in an editor that reveals hidden Unicode characters.
Learn more about bidirectional Unicode characters
Original file line number | Diff line number | Diff line change |
---|---|---|
@@ -0,0 +1,42 @@ | ||
# Image segmentation with SAM2 and OpenVINO™ | ||
|
||
|
||
Segmentation - identifying which image pixels belong to an object - is a core task in computer vision and is used in a broad array of applications, from analyzing scientific imagery to editing photos. But creating an accurate segmentation model for specific tasks typically requires highly specialized work by technical experts with access to AI training infrastructure and large volumes of carefully annotated in-domain data. Reducing the need for task-specific modeling expertise, training compute, and custom data annotation for image segmentation is the main goal of the Segment Anything project. | ||
|
||
Previously, to solve any kind of segmentation problem, there were two classes of approaches. The first, interactive segmentation, allowed for segmenting any class of object but required a person to guide the method by iterative refining a mask. The second, automatic segmentation, allowed for segmentation of specific object categories defined ahead of time (e.g., cats or chairs) but required substantial amounts of manually annotated objects to train (e.g., thousands or even tens of thousands of examples of segmented cats), along with the compute resources and technical expertise to train the segmentation model. Neither approach provided a general, fully automatic approach to segmentation. | ||
|
||
Segment Anything Model is a generalization of these two classes of approaches. It is a single model that can easily perform both interactive segmentation and automatic segmentation. | ||
|
||
[Segment Anything Model 2 (SAM 2)](https://ai.meta.com/sam2/) is a foundation model towards solving promptable visual segmentation in images and videos. It extend SAM to video by considering images as a video with a single frame. When SAM 2 is applied to images the model behaves like SAM. SAM 2 has all the capabilities of SAM on static images. The model first converts the image into an image embedding that allows high quality masks to be efficiently produced from a prompt. A promptable and light-weight mask decoder accepts a image embedding and prompts (if any) on the current image and outputs a segmentation mask. SAM 2 supports point, box, and mask prompts. | ||
|
||
|
||
This notebook shows an example of how to convert and use Segment Anything Model 2 in OpenVINO format, allowing it to run on a variety of platforms that support an OpenVINO. | ||
|
||
The notebooks demonstrates how to work with model in 2 modes: | ||
|
||
* Interactive segmentation mode: in this demonstration you can upload image/video and specify point related to object using [Gradio](https://gradio.app/) interface and as the result you get segmentation mask for specified point. | ||
The following image shows an example of the input text and the corresponding predicted image. | ||
 | ||
|
||
* Automatic segmentation mode: masks for the entire image can be generated by sampling a large number of prompts over an image. | ||
|
||
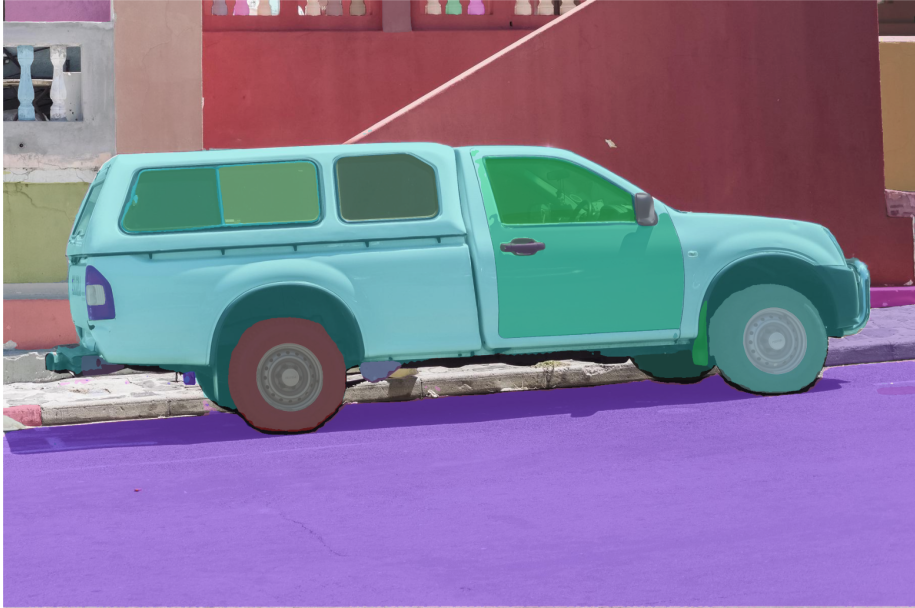 | ||
|
||
|
||
## Notebook Contents | ||
|
||
This notebook shows an example of how to convert and use Segment Anything Model 2 using OpenVINO | ||
|
||
Notebook contains the following steps: | ||
1. Convert PyTorch models to OpenVINO format. | ||
2. Run OpenVINO model in interactive segmentation mode. | ||
3. Run OpenVINO model in automatic mask generation mode. | ||
4. Run NNCF post-training optimization pipeline to compress the encoder of SAM | ||
|
||
|
||
## Installation Instructions | ||
|
||
This is a self-contained example that relies solely on its own code.</br> | ||
We recommend running the notebook in a virtual environment. You only need a Jupyter server to start. | ||
For details, please refer to [Installation Guide](../../README.md). | ||
<img referrerpolicy="no-referrer-when-downgrade" src="https://static.scarf.sh/a.png?x-pxid=5b5a4db0-7875-4bfb-bdbd-01698b5b1a77&file=notebooks/sam2-image-segmentation/README.md" /> |
File renamed without changes.
This file contains bidirectional Unicode text that may be interpreted or compiled differently than what appears below. To review, open the file in an editor that reveals hidden Unicode characters.
Learn more about bidirectional Unicode characters
Original file line number | Diff line number | Diff line change |
---|---|---|
@@ -0,0 +1,35 @@ | ||
import gradio as gr | ||
import numpy as np | ||
import cv2 | ||
|
||
|
||
def make_demo(segmenter): | ||
with gr.Blocks() as demo: | ||
with gr.Row(): | ||
input_img = gr.Image(label="Input", type="numpy", height=480, width=480) | ||
output_img = gr.Image(label="Selected Segment", type="numpy", height=480, width=480) | ||
|
||
def on_image_change(img): | ||
segmenter.set_image(img) | ||
return img | ||
|
||
def get_select_coords(img, evt: gr.SelectData): | ||
pixels_in_queue = set() | ||
h, w = img.shape[:2] | ||
pixels_in_queue.add((evt.index[0], evt.index[1])) | ||
out = img.copy() | ||
while len(pixels_in_queue) > 0: | ||
pixels = list(pixels_in_queue) | ||
pixels_in_queue = set() | ||
color = np.random.randint(0, 255, size=(1, 1, 3)) | ||
mask = segmenter.get_mask(pixels, img) | ||
mask_image = out.copy() | ||
mask_image[mask.squeeze(-1)] = color | ||
out = cv2.addWeighted(out.astype(np.float32), 0.7, mask_image.astype(np.float32), 0.3, 0.0) | ||
out = out.astype(np.uint8) | ||
return out | ||
|
||
input_img.select(get_select_coords, [input_img], output_img) | ||
input_img.upload(on_image_change, [input_img], [input_img]) | ||
|
||
return demo |
This file contains bidirectional Unicode text that may be interpreted or compiled differently than what appears below. To review, open the file in an editor that reveals hidden Unicode characters.
Learn more about bidirectional Unicode characters
Original file line number | Diff line number | Diff line change |
---|---|---|
@@ -0,0 +1,138 @@ | ||
import matplotlib.pyplot as plt | ||
|
||
from typing import Tuple | ||
|
||
import torch | ||
|
||
|
||
from copy import deepcopy | ||
from typing import Tuple | ||
from torchvision.transforms.functional import resize, to_pil_image | ||
|
||
import numpy as np | ||
|
||
np.random.seed(3) | ||
|
||
|
||
def show_mask(mask, ax): | ||
color = np.array([30 / 255, 144 / 255, 255 / 255, 0.6]) | ||
h, w = mask.shape[-2:] | ||
mask_image = mask.reshape(h, w, 1) * color.reshape(1, 1, -1) | ||
ax.imshow(mask_image) | ||
|
||
|
||
def show_points(coords, labels, ax, marker_size=375): | ||
pos_points = coords[labels == 1] | ||
neg_points = coords[labels == 0] | ||
ax.scatter( | ||
pos_points[:, 0], | ||
pos_points[:, 1], | ||
color="green", | ||
marker="*", | ||
s=marker_size, | ||
edgecolor="white", | ||
linewidth=1.25, | ||
) | ||
ax.scatter( | ||
neg_points[:, 0], | ||
neg_points[:, 1], | ||
color="red", | ||
marker="*", | ||
s=marker_size, | ||
edgecolor="white", | ||
linewidth=1.25, | ||
) | ||
|
||
|
||
def show_box(box, ax): | ||
x0, y0 = box[0], box[1] | ||
w, h = box[2] - box[0], box[3] - box[1] | ||
ax.add_patch(plt.Rectangle((x0, y0), w, h, edgecolor="green", facecolor=(0, 0, 0, 0), lw=2)) | ||
|
||
|
||
def show_masks(image, masks, point_coords=None, box_coords=None, input_labels=None, borders=True): | ||
for i, mask in enumerate(masks): | ||
plt.figure(figsize=(10, 10)) | ||
plt.imshow(image) | ||
show_mask(mask, plt.gca()) | ||
if point_coords is not None: | ||
assert input_labels is not None | ||
show_points(point_coords, input_labels, plt.gca()) | ||
if box_coords is not None: | ||
# boxes | ||
show_box(box_coords, plt.gca()) | ||
plt.axis("off") | ||
plt.show() | ||
|
||
|
||
class ResizeLongestSide: | ||
""" | ||
Resizes images to longest side 'target_length', as well as provides | ||
methods for resizing coordinates and boxes. Provides methods for | ||
transforming numpy arrays. | ||
""" | ||
|
||
def __init__(self, target_length: int) -> None: | ||
self.target_length = target_length | ||
|
||
def apply_image(self, image: np.ndarray) -> np.ndarray: | ||
""" | ||
Expects a numpy array with shape HxWxC in uint8 format. | ||
""" | ||
target_size = self.get_preprocess_shape(image.shape[0], image.shape[1], self.target_length) | ||
return np.array(resize(to_pil_image(image), target_size)) | ||
|
||
def apply_coords(self, coords: np.ndarray, original_size: Tuple[int, ...]) -> np.ndarray: | ||
""" | ||
Expects a numpy array of length 2 in the final dimension. Requires the | ||
original image size in (H, W) format. | ||
""" | ||
old_h, old_w = original_size | ||
new_h, new_w = self.get_preprocess_shape(original_size[0], original_size[1], self.target_length) | ||
coords = deepcopy(coords).astype(float) | ||
coords[..., 0] = coords[..., 0] * (new_w / old_w) | ||
coords[..., 1] = coords[..., 1] * (new_h / old_h) | ||
return coords | ||
|
||
def apply_boxes(self, boxes: np.ndarray, original_size: Tuple[int, ...]) -> np.ndarray: | ||
""" | ||
Expects a numpy array shape Bx4. Requires the original image size | ||
in (H, W) format. | ||
""" | ||
boxes = self.apply_coords(boxes.reshape(-1, 2, 2), original_size) | ||
return boxes.reshape(-1, 4) | ||
|
||
@staticmethod | ||
def get_preprocess_shape(oldh: int, oldw: int, long_side_length: int) -> Tuple[int, int]: | ||
""" | ||
Compute the output size given input size and target long side length. | ||
""" | ||
scale = long_side_length * 1.0 / max(oldh, oldw) | ||
newh, neww = oldh * scale, oldw * scale | ||
neww = int(neww + 0.5) | ||
newh = int(newh + 0.5) | ||
return (newh, neww) | ||
|
||
|
||
def preprocess_image(image: np.ndarray, resizer): | ||
resized_image = resizer.apply_image(image) | ||
resized_image = (resized_image.astype(np.float32) - [123.675, 116.28, 103.53]) / [ | ||
58.395, | ||
57.12, | ||
57.375, | ||
] | ||
resized_image = np.expand_dims(np.transpose(resized_image, (2, 0, 1)).astype(np.float32), 0) | ||
|
||
# Pad | ||
h, w = resized_image.shape[-2:] | ||
padh = 1024 - h | ||
padw = 1024 - w | ||
x = np.pad(resized_image, ((0, 0), (0, 0), (0, padh), (0, padw))) | ||
return x | ||
|
||
|
||
def postprocess_masks(masks: np.ndarray, orig_size, resizer): | ||
size_before_pad = resizer.get_preprocess_shape(orig_size[0], orig_size[1], masks.shape[-1]) | ||
masks = masks[..., : int(size_before_pad[0]), : int(size_before_pad[1])] | ||
masks = torch.nn.functional.interpolate(torch.from_numpy(masks), size=orig_size, mode="bilinear", align_corners=False).numpy() | ||
return masks |
37 changes: 24 additions & 13 deletions
37
...t-anything/segment-anything-2-image.ipynb → ...gmentation/segment-anything-2-image.ipynb
Large diffs are not rendered by default.
Oops, something went wrong.
This file contains bidirectional Unicode text that may be interpreted or compiled differently than what appears below. To review, open the file in an editor that reveals hidden Unicode characters.
Learn more about bidirectional Unicode characters
Original file line number | Diff line number | Diff line change |
---|---|---|
@@ -0,0 +1,30 @@ | ||
# Object masks from prompts with SAM and OpenVINO™ | ||
|
||
|
||
Segmentation - identifying which image pixels belong to an object - is a core task in computer vision and is used in a broad array of applications, from analyzing scientific imagery to editing photos. But creating an accurate segmentation model for specific tasks typically requires highly specialized work by technical experts with access to AI training infrastructure and large volumes of carefully annotated in-domain data. Reducing the need for task-specific modeling expertise, training compute, and custom data annotation for image segmentation is the main goal of the Segment Anything project. | ||
|
||
[Segment Anything Model 2 (SAM 2)](https://ai.meta.com/sam2/) is a foundation model towards solving promptable visual segmentation in images and videos. It extend SAM to video by considering images as a video with a single frame. The SAM 2 model extends the promptable capability of SAM to the video domain by adding a per session memory module that captures information about the target object in the video. This allows SAM 2 to track the selected object throughout all video frames, even if the object temporarily disappears from view, as the model has context of the object from previous frames. SAM 2 also supports the ability to make corrections in the mask prediction based on additional prompts on any frame. SAM 2’s streaming architecture—which processes video frames one at a time—is also a natural generalization of SAM to the video domain. When SAM 2 is applied to images, the memory module is empty and the model behaves like SAM. | ||
|
||
The model design is a simple transformer architecture with streaming memory for real-time video processing. The model is built a model-in-the-loop data engine, which improves model and data via user interaction, to collect SA-V dataset, the largest video segmentation dataset to date. SAM 2 provides strong performance across a wide range of tasks and visual domains. | ||
This notebook shows an example of how to convert and use Segment Anything Model 2 in OpenVINO format, allowing it to run on a variety of platforms that support an OpenVINO. | ||
|
||
* Interactive segmentation mode: in this demonstration you can upload image/video and specify point related to object using [Gradio](https://gradio.app/) interface and as the result you get segmentation mask for specified point. | ||
The following image shows an example of the input text and the corresponding predicted image. | ||
 | ||
|
||
|
||
## Notebook Contents | ||
|
||
This notebook shows an example of how to convert and use Segment Anything Model using OpenVINO | ||
|
||
Notebook contains the following steps: | ||
1. Convert PyTorch models to OpenVINO format. | ||
2. Run OpenVINO model in interactive segmentation mode. | ||
|
||
|
||
## Installation Instructions | ||
|
||
This is a self-contained example that relies solely on its own code.</br> | ||
We recommend running the notebook in a virtual environment. You only need a Jupyter server to start. | ||
For details, please refer to [Installation Guide](../../README.md). | ||
<img referrerpolicy="no-referrer-when-downgrade" src="https://static.scarf.sh/a.png?x-pxid=5b5a4db0-7875-4bfb-bdbd-01698b5b1a77&file=notebooks/sam2-video-segmentation/README.md" /> |
This file contains bidirectional Unicode text that may be interpreted or compiled differently than what appears below. To review, open the file in an editor that reveals hidden Unicode characters.
Learn more about bidirectional Unicode characters
Original file line number | Diff line number | Diff line change |
---|---|---|
@@ -0,0 +1,49 @@ | ||
import gradio as gr | ||
import numpy as np | ||
import cv2 | ||
|
||
|
||
def make_video_demo(segmenter, sample_path): | ||
with gr.Blocks() as demo: | ||
with gr.Row(): | ||
with gr.Column(): | ||
input_video = gr.Video(label="Video") | ||
coordinates = gr.Textbox(label="Coordinates") | ||
labels = gr.Textbox(label="Labels") | ||
submit_btn = gr.Button(value="Segment") | ||
with gr.Column(): | ||
output_video = gr.Video(label="Output video") | ||
|
||
def on_video_change(video): | ||
segmenter.set_video(video) | ||
return video | ||
|
||
def segment_video(video, coordinates_txt, labels_txt): | ||
coordinates_np = [] | ||
for coords in coordinates_txt.split(";"): | ||
temp = [float(numb) for numb in coords.split(",")] | ||
if len(temp) == 4: | ||
box_coords = np.array(temp).reshape(2, 2) | ||
coordinates_np.append(box_coords) | ||
else: | ||
coordinates_np.append(temp) | ||
|
||
labels_np = [] | ||
for l in labels_txt.split(","): | ||
labels_np.append(int(l)) | ||
segmenter.set_video(video) | ||
segmenter.add_new_points_or_box(coordinates_np, labels_np) | ||
segmenter.propagate_in_video() | ||
video_out_path = segmenter.save_as_video() | ||
|
||
return video_out_path | ||
|
||
submit_btn.click(segment_video, inputs=[input_video, coordinates, labels], outputs=[output_video]) | ||
input_video.upload(on_video_change, [input_video], [input_video]) | ||
|
||
examples = gr.Examples( | ||
examples=[[sample_path / "coco.mp4", "430, 130; 500, 100", "1, 1"], [sample_path / "coco.mp4", "380, 75, 530, 260", "2, 3"]], | ||
inputs=[input_video, coordinates, labels], | ||
) | ||
|
||
return demo |
Oops, something went wrong.