-
Notifications
You must be signed in to change notification settings - Fork 0
/
PoissonRegressionModels.Rmd
206 lines (155 loc) · 9.89 KB
/
PoissonRegressionModels.Rmd
1
2
3
4
5
6
7
8
9
10
11
12
13
14
15
16
17
18
19
20
21
22
23
24
25
26
27
28
29
30
31
32
33
34
35
36
37
38
39
40
41
42
43
44
45
46
47
48
49
50
51
52
53
54
55
56
57
58
59
60
61
62
63
64
65
66
67
68
69
70
71
72
73
74
75
76
77
78
79
80
81
82
83
84
85
86
87
88
89
90
91
92
93
94
95
96
97
98
99
100
101
102
103
104
105
106
107
108
109
110
111
112
113
114
115
116
117
118
119
120
121
122
123
124
125
126
127
128
129
130
131
132
133
134
135
136
137
138
139
140
141
142
143
144
145
146
147
148
149
150
151
152
153
154
155
156
157
158
159
160
161
162
163
164
165
166
167
168
169
170
171
172
173
174
175
176
177
178
179
180
181
182
183
184
185
186
187
188
189
190
191
192
193
194
195
196
197
198
199
200
201
202
203
204
205
206
---
title: "Poisson regression models (Table 2 of manuscript)"
author: "Sara Venkatraman"
date: "1/12/2021"
output: rmarkdown::github_document
---
```{r setup, include=FALSE}
knitr::opts_chunk$set(echo = TRUE)
```
#### Model setup
First we run a script which reads the syndromic surveillance data, and we also load a few libraries.
```{r, message=FALSE, warning=FALSE}
# Load script which reads the syndromic surveillance data and sets up the design matrices used for modeling
source("ModelingSetup.R")
# Packages for obtaining robust standard errors and VIFs
library(lmtest); library(sandwich); library(car); library(MASS)
# Packages for spatiotemporal modeling
library(maptools); library(spdep); library(INLA)
# Packages for plotting and printing tables
library(ggplot2); library(gridExtra); library(knitr)
```
Now we read in a zip code-level NYC shapefile that will later enable us to construct spatiotemporal models of case counts over time and over 173 zip codes.
```{r, echo=FALSE, warning=FALSE, message=FALSE}
# Read NYC shapefiles
NYC <- readShapePoly("../Paper 1 Surveillance Analysis/Spatiotemporal Model Files/tl_2010_36_zcta510NYC.shp")
NYC2 <- st_read("../Paper 1 Surveillance Analysis/Spatiotemporal Model Files/tl_2010_36_zcta510NYC.shp")
NYC <- NYC[NYC$ZCTA5CE10 %in% allZipcodes,]
NYC2 <- NYC2[NYC2$ZCTA5CE10 %in% allZipcodes,]
# Obtain list of each zipcode's neighbors and the coordinates of their centroids
zctaNeighbors <- poly2nb(NYC)
zctaCoords <- st_coordinates(st_centroid(st_geometry(NYC2)))
# Save/view the zipcode adjacency list
nb2INLA("NYC.graph", zctaNeighbors)
NYCadj <- paste("nyc.graph")
# Reorder NYC surveillance data to match the order of the zipcodes in shapefile
NYCgeo <- attr(NYC, "data")
shpOrder <- match(NYCgeo$ZCTA5CE10, allZipcodes)
zctaOrder <- allZipcodes[shpOrder]
```
The next few lines of code produce a dataframe ("design matrix") of the following form. Below, "Case count" refers to the suspected cases, i.e. the total number of ILI + pneumonia emergency department presentations observed on that day. In this dataframe, both overcrowdedness and multigenerational housing are binned into quartiles.
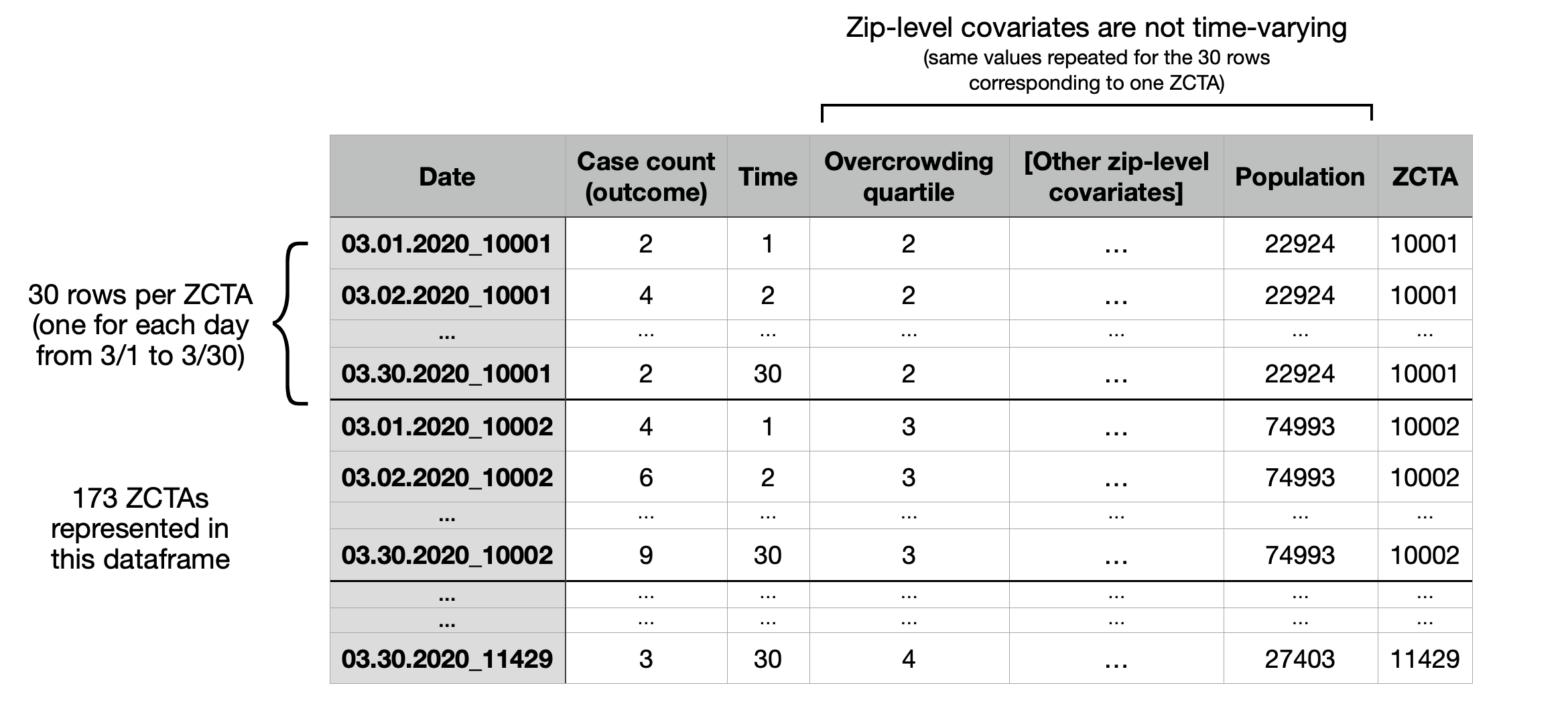{width="70%"}
```{r}
# Design matrix construction
designResponse.ili <- Concatenate.Zipcode.Data(zctaOrder, "influenza", "2020-03-16", variablesToDiscretize=c("PctOvercrowded", "PctMultigen"), quartile=T)
designResponse.pneu <- Concatenate.Zipcode.Data(zctaOrder, "pneumonia", "2020-03-16", variablesToDiscretize=c("PctOvercrowded", "PctMultigen"), quartile=T)
designResponse <- cbind(designResponse.ili$Count + designResponse.pneu$Count, designResponse.ili[,-1])
colnames(designResponse)[1] <- "Count"; remove(designResponse.ili); remove(designResponse.pneu)
# Get sum of essential employment percentages
designResponse$PctEssEmpl <- rowSums(designResponse[,18:23])
```
Define functions for neatly printing the model coefficients and confidence intervals. The first function applies to GLMs and the second applies to INLA models.
```{r}
Print.Model.Results.GLM <- function(modelGLM, numDecimal) {
# Get coefficient estimates and confidence intervals. Combine them (along with the)
# p-value) into one table, called 'modelResults' - results rounded to 'numDecimal'
modelCoef <- coeftest(modelGLM)
modelCI <- coefci(modelGLM, vcov=vcovHC(modelGLM, type="HC3"))
modelResults <- cbind(round(exp(modelCoef[,1]), numDecimal),
modelCoef[,4],
round(exp(modelCI[,1:2]), numDecimal))
colnames(modelResults)[1:2] <- c("exp(Estimate)", "p-value")
# Create a 1-column table called 'resultsSummary' that stores model results in
# the following format: "exp(Estimate), (ciLower, ciUpper)"
resultsSummary <- matrix("", nrow=nrow(modelResults), ncol=1)
rownames(resultsSummary) <- rownames(modelResults)
for(i in 1:nrow(modelResults)) {
string.i <- paste(modelResults[i,1], " (", modelResults[i,3], ", ", modelResults[i,4], ")", sep="")
resultsSummary[i,1] <- string.i
}
resultsSummary <- resultsSummary[c(3:nrow(resultsSummary), 2, 1), ]
return(modelResults)
}
Print.Model.Results.INLA <- function(modelINLA, numDecimal) {
# Get coefficient estimates and confidence intervals. Combine them (along with the)
# p-value) into one table, called 'modelResults' - results rounded to 'numDecimal'
modelResults <- cbind(round(exp(model5.INLA$summary.fixed[,1]), numDecimal),
model5.INLA$summary.fixed[,2],
round(exp(model5.INLA$summary.fixed[,-c(1:2,4,7)]), numDecimal))
colnames(modelResults)[1:2] <- c("exp(Estimate)", "SD")
# Create a 1-column table called 'resultsSummary' that stores model results in
# the following format: "exp(Estimate), (ciLower, ciUpper)"
resultsSummary <- matrix("", nrow=nrow(modelResults), ncol=1)
rownames(resultsSummary) <- rownames(modelResults)
for(i in 1:nrow(modelResults)) {
string.i <- paste(modelResults[i,1], " (", modelResults[i,3], ", ", modelResults[i,4], ")", sep="")
resultsSummary[i,1] <- string.i
}
resultsSummary <- resultsSummary[c(3:nrow(resultsSummary), 2, 1), ]
return(modelResults)
}
```
#### Model 1: Housing-related exposure covariates only (quasi-Poisson GLM)
```{r}
model1.ILIpneu <- glm(Count ~ Time + PctOvercrowded + PctMultigen + offset(log(Population/10000)), family=quasipoisson, data=designResponse)
# Check variance inflation factors. None need to be removed (based on VIF > 10 criterion)
kable(vif(model1.ILIpneu))
# Print model results
kable(Print.Model.Results.GLM(model1.ILIpneu, 2))
```
#### Model 2: Add clinical risk factors for COVID-19 to model 1
```{r}
model2.ILIpneu <- glm(Count ~ Time + PctOvercrowded + PctMultigen + BPHIGH_CrudePrev + DIABETES_CrudePrev + CHD_CrudePrev + OBESITY_CrudePrev + COPD_CrudePrev + CSMOKING_CrudePrev + offset(log(Population/10000)), family=quasipoisson, data=designResponse)
# Check variance inflation factors. COPD has the largest VIF.
kable(vif(model2.ILIpneu))
# Re-fit model without COPD
model2.ILIpneu <- glm(Count ~ Time + PctOvercrowded + PctMultigen + BPHIGH_CrudePrev + DIABETES_CrudePrev + CHD_CrudePrev + OBESITY_CrudePrev + CSMOKING_CrudePrev + offset(log(Population/10000)), family=quasipoisson, data=designResponse)
# Check variance inflation factors. Hypertension now has the largest VIF.
kable(vif(model2.ILIpneu))
# Re-fit model without COPD and hypertension
model2.ILIpneu <- glm(Count ~ Time + PctOvercrowded + PctMultigen + DIABETES_CrudePrev + CHD_CrudePrev + OBESITY_CrudePrev + CSMOKING_CrudePrev + offset(log(Population/10000)), family=quasipoisson, data=designResponse)
# Check variance inflation factors. No more variables need to be removed.
kable(vif(model2.ILIpneu))
# Print model results
kable(Print.Model.Results.GLM(model2.ILIpneu, 2))
```
#### Model 3: Add socioeconomic covariates to model 1
```{r}
model3.ILIpneu <- glm(Count ~ Time + PctOvercrowded + PctMultigen + PctWhite + PctBelowPovThresh + MedianIncome + PctEssEmpl + PopDensity + offset(log(Population/10000)), family=quasipoisson, data=designResponse)
# Check variance inflation factors. No variables need to be removed.
kable(vif(model3.ILIpneu))
# Print model results
kable(Print.Model.Results.GLM(model3.ILIpneu, 2))
```
#### Model 4: Add both clinical and socioeconomic covariates to model 1
```{r}
model4.ILIpneu <- glm(Count ~ Time + PctOvercrowded + PctMultigen + BPHIGH_CrudePrev + DIABETES_CrudePrev + CHD_CrudePrev + OBESITY_CrudePrev + COPD_CrudePrev + CSMOKING_CrudePrev + PctWhite + PctBelowPovThresh + MedianIncome + PctEssEmpl + PopDensity + offset(log(Population/10000)), family=quasipoisson, data=designResponse)
# Check variance inflation factors. COPD has the largest VIF.
kable(vif(model4.ILIpneu))
# Re-fit model without COPD
model4.ILIpneu <- glm(Count ~ Time + PctOvercrowded + PctMultigen + BPHIGH_CrudePrev + DIABETES_CrudePrev + CHD_CrudePrev + OBESITY_CrudePrev + CSMOKING_CrudePrev + PctWhite + PctBelowPovThresh + MedianIncome + PctEssEmpl + PopDensity + offset(log(Population/10000)), family=quasipoisson, data=designResponse)
# Check variance inflation factors. Hypertension now has the largest VIF.
kable(vif(model4.ILIpneu))
# Re-fit model without COPD and hypertension
model4.ILIpneu <- glm(Count ~ Time + PctOvercrowded + PctMultigen + DIABETES_CrudePrev + CHD_CrudePrev + OBESITY_CrudePrev + CSMOKING_CrudePrev + PctWhite + PctBelowPovThresh + MedianIncome + PctEssEmpl + PopDensity + offset(log(Population/10000)), family=quasipoisson, data=designResponse)
# Check variance inflation factors. Diabetes now has the largest VIF.
kable(vif(model4.ILIpneu))
# Re-fit model without COPD, hypertension, and diabetes
model4.ILIpneu <- glm(Count ~ Time + PctOvercrowded + PctMultigen + CHD_CrudePrev + OBESITY_CrudePrev + CSMOKING_CrudePrev + PctWhite + PctBelowPovThresh + MedianIncome + PctEssEmpl + PopDensity + offset(log(Population/10000)), family=quasipoisson, data=designResponse)
# Check variance inflation factors. No more variables need to be removed.
kable(vif(model4.ILIpneu))
# Print model results
kable(Print.Model.Results.GLM(model4.ILIpneu, 2))
```
#### Model 5: Bayesian spatiotemporal model, using model 4 covariates
```{r}
# Add zip code ID number to design matrix (needed for spatial and temporal random effects)
zipcodeID <- sort(rep(1:length(allZipcodes), 30))
designResponse$ZipID <- zipcodeID
designResponse$ZipID2 <- zipcodeID
# Construct spatiotemporal model using same set of covariates in (reduced) model 4
model5.INLAformula <- Count ~ 1 + f(ZipID, model="bym", offset(Population/10000), graph=NYCadj) + f(ZipID2, Time, model="rw1") + Time + PctOvercrowded + PctMultigen + CHD_CrudePrev + OBESITY_CrudePrev + CSMOKING_CrudePrev + PctWhite + PctBelowPovThresh + MedianIncome + PctEssEmpl + PopDensity
model5.INLA <- inla(model5.INLAformula, family="poisson", data=designResponse, control.compute=list(dic=TRUE,cpo=TRUE))
# Print model results
kable(Print.Model.Results.INLA(model5.INLA, 2))
```